The realm of e-commerce has experienced exponential growth in recent years, fundamentally altering the landscape of retail and customer interaction. This surge in online shopping has underscored the importance of comprehensively understanding customer value for businesses. Central to this understanding is the concept of Customer Lifetime Value (CLV), a metric that estimates the total revenue a business can expect from a single customer account throughout their relationship with the company. CLV is not just a reflection of past behavior; it is a crucial predictor of future profitability, enabling businesses to make more informed decisions about marketing, sales strategies, and customer service.
The significance of CLV in e-commerce cannot be overstated. It allows companies to identify the most valuable customers and tailor their efforts to retain them. Moreover, understanding CLV helps in optimizing resource allocation, ensuring that marketing and customer acquisition costs are effectively balanced against the anticipated revenue from each customer.
In this context, predictive analytics emerges as a pivotal tool. It involves using data, statistical algorithms, and machine learning techniques to identify the likelihood of future outcomes based on historical data. For calculating CLV, predictive analytics can analyze vast amounts of data from various customer interactions, encompassing purchase history, browsing behavior, and engagement with marketing campaigns. This analysis can provide insights into customer preferences and behavior patterns, enabling businesses to forecast future purchasing actions and the resultant revenue.
The integration of predictive analytics in determining CLV represents a strategic advancement in e-commerce. It facilitates a more dynamic and personalized approach to customer relationship management, paving the way for enhanced customer experiences and sustained business growth. This paper will delve into the methodologies of predictive analytics for calculating CLV, its implementation challenges, and the potential it holds for revolutionizing customer value assessment in e-commerce.
Table of Contents
Understanding Customer Lifetime Value (CLV)
Customer Lifetime Value (CLV) is a pivotal metric in e-commerce, offering a comprehensive view of the worth of a customer to a business over the entire duration of their relationship. Understanding CLV is crucial for businesses seeking to maximize profitability and foster long-term customer relationships. The calculation of CLV typically involves several key components:
Customer Lifespan: This element refers to the duration of the relationship between the customer and the business. It is an estimate of how long a customer is expected to continue making purchases. A longer customer lifespan generally indicates greater loyalty and a higher CLV.
Average Purchase Value: This metric represents the average amount spent by a customer in a typical transaction. To calculate it, businesses divide the total revenue earned from a customer by the number of purchases they have made over a given period.
Purchase Frequency: This component assesses how often a customer makes a purchase within a specified time frame. It helps businesses understand the regularity of a customer’s engagement and spending.
The significance of CLV extends beyond mere transactional value; it plays a critical role in several strategic areas:
Customer Segmentation: By calculating CLV, businesses can segment their customers into various groups based on their value. This segmentation allows for more targeted marketing efforts, as companies can focus on retaining high-value customers and nurturing those with potential for increased profitability.
Retention Strategies: Understanding CLV helps in devising effective customer retention strategies. By identifying the characteristics of high-value customers, businesses can tailor their services and communications to meet these customers’ specific needs and preferences, thereby increasing the likelihood of retaining them.
Profitability Analysis: CLV is a crucial factor in analyzing the overall profitability of a company’s customer base. It helps in evaluating the return on investment for marketing and customer acquisition efforts. By focusing on customers with a higher CLV, businesses can allocate resources more efficiently and enhance their profit margins.
CLV serves as a guiding metric for businesses in e-commerce, steering decisions related to marketing, customer service, and strategic planning. It enables a more nuanced understanding of the customer base, highlighting the long-term value of customer relationships over short-term gains. This understanding is essential for businesses aiming to thrive in the competitive landscape of e-commerce.
Role of Predictive Analytics in CLV
Predictive analytics represents a significant evolution in the field of data analysis, particularly in its application to Customer Lifetime Value (CLV) in e-commerce. Differing fundamentally from traditional analytical methods, predictive analytics does not merely report what has happened or what is happening. Instead, it focuses on forecasting what might happen in the future. This forward-looking approach is especially useful in calculating and maximizing CLV.
Overview of Predictive Analytics: Traditional analytics often involve descriptive methods that provide insights into past performance, such as summarizing past sales data or customer behaviors. Predictive analytics, however, utilizes advanced techniques like statistical algorithms, data mining, and machine learning to analyze current and historical facts to make predictions about future events. In the context of CLV, it helps in predicting how customers will behave over time, what their future purchasing patterns might be, and how long they are likely to remain active customers.
Predictive Models in CLV Calculations:
Regression Models: These are statistical models used to estimate the relationships among variables. In the context of CLV, regression models can be used to understand how various factors like purchase frequency, product preferences, customer demographics, and previous buying behaviors are likely to impact future spending.
Cohort Analysis: This involves grouping customers into cohorts based on shared characteristics or experiences within a defined time-span. For CLV, cohorts can be based on the time of the first purchase, product types purchased, or customer demographics. Predictive analytics can then be applied to these cohorts to forecast their future behavior and CLV.
Machine Learning Algorithms: Machine learning offers a more advanced approach to predicting CLV. Algorithms can be trained on large datasets, learning from patterns in customer behavior. These algorithms, which include decision trees, random forests, and neural networks, can adapt and improve over time, providing increasingly accurate predictions about CLV as more data becomes available.
Predictive analytics transforms CLV from a static historical metric into a dynamic tool that can anticipate future customer behavior and value. By leveraging these predictive models, businesses can tailor their strategies to individual customer needs and preferences, allocate resources more efficiently, and ultimately drive higher profitability through more informed decision-making. This approach marks a significant shift in how businesses understand and interact with their customers, offering a more nuanced and proactive approach to customer relationship management.
Data-Driven Insights for CLV Enhancement
The effectiveness of predictive analytics in enhancing Customer Lifetime Value (CLV) hinges critically on the quality and comprehensiveness of the data used. Quality data acts as the foundation upon which accurate predictions and meaningful insights are built, enabling businesses to make informed decisions that positively impact CLV.
Importance of Quality Data in Predictive Analytics:
- High-quality data ensures that the predictive models are reliable and the forecasts they generate are valid. This includes data that is accurate, complete, timely, and relevant.
- Inconsistent or poor-quality data can lead to inaccurate predictions, potentially resulting in misguided strategies and decisions.
- Regular data cleansing and validation are crucial to maintain the integrity of the predictive models over time.
Types of Data Used for CLV Prediction:
- Demographic Data: Includes age, gender, income level, education, and occupation. This data helps in segmenting customers and understanding the purchasing behavior of different demographic groups.
- Transactional Data: Encompasses purchase history, amount spent, frequency of purchases, and types of products bought. This data is crucial in calculating the historical value of a customer and in predicting future purchasing patterns.
- Behavioral Data: Relates to how customers interact with a business, including website browsing patterns, responses to marketing campaigns, and engagement on social media platforms. Behavioral data provides insights into customer preferences and engagement levels.
- Psychographic Data: Concerns lifestyle, interests, attitudes, and values. Though more subjective, this data can be instrumental in understanding the motivations behind customer purchases.
Case Studies/Examples of Successful CLV Prediction and Enhancement:
- E-commerce Retailer: An online retailer used machine learning algorithms to analyze transactional and behavioral data. By identifying patterns in purchase history and customer engagement, the retailer was able to predict CLV with greater accuracy, enabling them to tailor marketing campaigns to high-value customers and increase overall profitability.
- Subscription-Based Service: A subscription service utilized demographic and transactional data to segment its customer base. Through predictive analytics, they identified which segments had the highest CLV and tailored their service offerings to these segments, resulting in increased retention and higher CLV.
- Retail Banking: A bank applied predictive analytics to transactional and demographic data to forecast the CLV of their clients. The insights gained allowed them to customize their financial products and advisory services, enhancing customer satisfaction and loyalty, and thereby increasing the CLV.
These examples underscore the transformative potential of data-driven insights in CLV enhancement. By leveraging quality data across various dimensions, businesses can not only predict CLV with greater accuracy but also implement targeted strategies to maximize the value derived from their customer relationships. The key lies in the intelligent integration of data analytics into strategic decision-making processes, ensuring that customer value is continuously optimized.
Challenges and Considerations
Implementing predictive analytics for Customer Lifetime Value (CLV) enhancement comes with its set of challenges and ethical considerations. Addressing these effectively is crucial for maintaining the integrity of the process and ensuring customer trust and satisfaction.
Common Challenges in Implementing Predictive Analytics:
- Data Quality: Ensuring the accuracy, completeness, and relevance of data is a significant challenge. Inaccurate or incomplete data can lead to faulty predictions. Businesses must establish robust data collection and management practices to maintain high-quality data.
- Privacy Concerns: With increasing awareness and regulation around data privacy (such as GDPR in Europe), handling customer data responsibly is a major concern. Businesses must ensure compliance with legal standards and implement strong data security measures to protect customer information.
- Model Accuracy: Developing predictive models that accurately forecast CLV is complex. These models must be continuously tested and updated to adapt to changing customer behaviors and market conditions. Overreliance on historical data without considering current trends can also lead to inaccurate predictions.
Ethical Considerations in Data Usage and Customer Privacy:
- Transparency: Businesses should be transparent with customers about what data is being collected and how it is being used. This includes clear communication through privacy policies and consent forms.
- Data Minimization: Collect only the data that is necessary for the intended purpose. Excessive data collection without a clear purpose can lead to ethical and legal complications.
- Bias and Fairness: Predictive models can inadvertently perpetuate biases present in the training data, leading to unfair outcomes for certain customer groups. Regular audits and adjustments of models are necessary to ensure fairness and unbiased decision-making.
- Customer Control: Customers should have control over their data, including the ability to access, correct, and delete their information. Respecting customer autonomy in data decisions is both an ethical and legal imperative.
Addressing these challenges and ethical considerations requires a balanced approach, blending technical, legal, and ethical expertise. Businesses must invest in robust data management and analytics infrastructure, adhere to legal and ethical standards, and maintain transparency and fairness in their data practices. This not only ensures compliance and risk mitigation but also fosters customer trust and loyalty, which are essential for long-term success in e-commerce.
Integrating Predictive Analytics into E-commerce Strategy
Effectively incorporating predictive analytics into an e-commerce strategy, particularly for enhancing Customer Lifetime Value (CLV), necessitates a holistic approach that aligns data-driven insights with business objectives. Below are strategies for integrating CLV predictions into broader e-commerce initiatives:
Personalized Marketing:
- Utilize CLV predictions to tailor marketing efforts. Customers with higher predicted CLVs can be targeted with premium offers, loyalty programs, and personalized recommendations.
- Implement targeted email campaigns and personalized content on websites and apps based on customer preferences and past purchasing behavior.
Dynamic Pricing:
- Employ CLV data to inform dynamic pricing strategies. For high-value customers, exclusive discounts or special pricing can be offered to encourage repeat purchases and increase their lifetime value.
- Conversely, for customers with lower CLVs, a different pricing strategy might be more effective, such as bundling products or offering incentives for bulk purchases.
Customer Experience Enhancement:
- Enhance customer service by understanding customer needs and expectations through CLV insights. High-CLV customers might benefit from premium support services like personalized shopping assistance or priority customer service.
- Use CLV data to identify and rectify pain points in the customer journey, thereby improving overall customer satisfaction and retention.
Role of Technology and Tools:
- CRM Systems: Customer Relationship Management (CRM) systems play a critical role in supporting predictive analytics. They can store and manage vast amounts of customer data, integrate with analytics tools to process this data, and provide insights into customer behavior and CLV.
- Data Analytics Platforms: Advanced analytics platforms, which often incorporate AI and machine learning capabilities, are essential for processing large datasets and generating accurate CLV predictions. These tools can automate data analysis, identify trends, and provide actionable insights.
- Integration with E-commerce Platforms: Ensuring that predictive analytics tools are seamlessly integrated with existing e-commerce platforms and databases is crucial. This integration allows for real-time data analysis and the immediate application of insights into e-commerce strategies.
- Cloud Computing: Leveraging cloud computing can provide the necessary scalability and flexibility for data storage and analytics processes, especially when dealing with large volumes of data and complex predictive models.
By integrating predictive analytics into these areas, e-commerce businesses can create a more dynamic, responsive, and customer-centric approach. It’s about moving beyond one-size-fits-all strategies to a more nuanced understanding of customer value, using technology and data to drive decisions and create personalized experiences that resonate with different customer segments. This strategic integration not only enhances the effectiveness of marketing and sales efforts but also contributes significantly to building long-term customer relationships and loyalty.
Future Trends and Evolutions in Predictive Analytics and CLV
The landscape of predictive analytics and Customer Lifetime Value (CLV) modeling is continuously evolving, driven by technological advancements and changing market dynamics. The future of these fields is likely to be shaped by several emerging trends:
Advancements in AI and Machine Learning:
- The integration of Artificial Intelligence (AI) and advanced machine learning techniques in predictive analytics is set to become more sophisticated. This includes the use of deep learning algorithms that can analyze more complex data patterns and provide more accurate predictions of CLV.
- AI-driven predictive models will increasingly handle unstructured data (like social media content, customer reviews, and voice recordings) to glean deeper insights into customer behavior and preferences.
Real-Time Analytics:
- The ability to analyze data in real-time will significantly enhance CLV modeling. Real-time analytics allows businesses to respond promptly to customer actions, offering immediate personalization in marketing and customer interactions.
- This shift will enable a more dynamic approach to CLV optimization, where customer value is constantly updated and strategies are adjusted in response to the latest data.
Integration of IoT and Predictive Analytics:
- The Internet of Things (IoT) offers a wealth of data from connected devices. Integrating this data into CLV models could provide a more holistic view of customer behavior and preferences.
- Predictive models could use IoT data to understand customer usage patterns, lifestyle choices, and even predict future needs, further personalizing the customer experience.
Enhanced Data Privacy and Ethics:
- As predictive analytics becomes more prevalent, there will be an increased focus on data privacy and ethical considerations. This may involve advanced techniques for anonymizing data and algorithms designed to ensure fairness and avoid bias.
- Regulatory compliance will become more integrated into predictive models, ensuring that CLV strategies align with evolving data protection laws.
Predictions on CLV Modeling and E-commerce:
- CLV modeling will likely become more granular, moving beyond broad segmentations to individualized predictions. This will enable e-commerce businesses to tailor their strategies to the unique value and needs of each customer.
- The use of predictive analytics in CLV will increasingly become a competitive differentiator in e-commerce. Businesses that can accurately predict and enhance CLV will gain a significant advantage in customer acquisition and retention.
- There will be a growing emphasis on predictive models that not only forecast CLV but also recommend specific actions and strategies to maximize it.
The future of predictive analytics and CLV in e-commerce is poised for significant transformations. These advancements will offer businesses deeper insights, greater personalization capabilities, and more effective strategies for maximizing customer value. As a result, the ability to adapt to and leverage these emerging trends will be crucial for businesses looking to thrive in the dynamic world of e-commerce.
Case Studies on Predictive Analytics for Customer Lifetime Value in E-commerce
There are several notable case studies that highlight the effective use of predictive analytics for Customer Lifetime Value (CLV) in the e-commerce sector. These case studies demonstrate how businesses can leverage data and predictive models to enhance customer understanding, tailor marketing strategies, and ultimately drive profitability. Here are a few examples:
Amazon: Personalized Recommendations
Amazon is renowned for its use of predictive analytics to offer personalized product recommendations. By analyzing customer purchase history, browsing behavior, and product preferences, Amazon’s algorithms predict what customers might be interested in next, thereby enhancing the shopping experience and increasing customer lifetime value.
This approach not only drives repeat purchases but also enhances customer engagement, contributing to Amazon’s strong customer loyalty and high CLV.
Netflix: Content Tailoring and Customer Retention
Netflix’s use of predictive analytics extends beyond recommending shows and movies. It also informs their content creation and acquisition strategies. By analyzing detailed customer viewing patterns and preferences, Netflix can predict which types of content are likely to retain customers, thereby increasing CLV.
This strategy has been instrumental in Netflix’s ability to maintain a loyal customer base, despite increasing competition in the streaming sector.
ASOS: Reducing Returns Through Size Prediction
Online fashion retailer ASOS implemented predictive analytics to tackle the issue of high return rates. By predicting the correct size for customers based on their previous purchases and returns data, ASOS was able to reduce the number of returns.
This not only improved customer satisfaction but also increased repeat purchase rates, directly impacting customer lifetime value.
Starbucks: Personalized Marketing
Starbucks uses predictive analytics to personalize its marketing efforts. By analyzing transaction data, Starbucks offers tailored promotions and recommendations through its mobile app. This personalization increases customer engagement and encourages frequent visits.
The effectiveness of this approach is evident in the increased usage of the Starbucks app and a corresponding rise in CLV.
eBay: Price Optimization
eBay employs predictive analytics for dynamic pricing, helping sellers determine optimal pricing points based on various factors including demand, time of day, and competition.
This strategy not only maximizes sales potential for sellers but also improves the overall customer experience by offering competitive prices, indirectly influencing CLV.
These case studies illustrate the diverse applications of predictive analytics in enhancing CLV. Whether it’s through personalized recommendations, content optimization, size prediction, tailored marketing, or dynamic pricing, these companies show how data-driven insights can lead to more effective business strategies and stronger customer relationships in the e-commerce domain.
Examples of Predictive Analytics for Customer Lifetime Value in E-commerce
In the realm of e-commerce, predictive analytics has been successfully applied to enhance Customer Lifetime Value (CLV) in various innovative ways. Here are some examples demonstrating how different e-commerce businesses have leveraged predictive analytics for CLV:
Personalized Product Recommendations:
Many e-commerce platforms, such as Amazon, use predictive analytics to offer personalized product recommendations. By analyzing past purchase history, browsing behavior, and customer ratings, these platforms predict what products a customer might be interested in, thereby increasing the likelihood of additional purchases, enhancing customer experience, and ultimately boosting CLV.
Optimizing Customer Segmentation:
E-commerce businesses utilize predictive analytics to segment their customers more effectively based on their predicted CLV. This segmentation enables targeted marketing strategies, where resources are focused on high-value segments to maximize returns. For example, a luxury goods retailer might identify and target high-income customer segments that exhibit a higher potential CLV.
Dynamic Pricing Models:
Predictive analytics aids in implementing dynamic pricing strategies where prices are adjusted in real-time based on demand, customer behavior, and market conditions. By predicting which customers are willing to pay more based on their purchasing patterns and price sensitivity, businesses can optimize pricing for different customer segments to enhance CLV.
Churn Prediction and Retention Strategies:
E-commerce companies use predictive models to identify customers who are at risk of churning. By analyzing factors such as purchase frequency, customer engagement, and feedback, businesses can implement targeted retention strategies to prevent churn and increase the lifetime value of at-risk customers.
Inventory Management and Demand Forecasting:
Predictive analytics helps e-commerce businesses in forecasting demand for various products. This foresight aids in efficient inventory management, ensuring that popular products are well-stocked and less popular ones aren’t over-ordered, thereby reducing costs and improving customer satisfaction, which positively impacts CLV.
Enhanced Customer Experience Through AI Chatbots:
AI-powered chatbots, using predictive analytics, can enhance customer service by predicting customer queries and providing instant, personalized responses. This improves the overall customer experience, leading to higher satisfaction and increased CLV.
Email Marketing Optimization:
Predictive analytics is used to optimize email marketing campaigns. By predicting the best times to send emails, personalizing email content based on customer preferences, and segmenting email lists, businesses can increase open rates and engagement, driving repeat purchases and improving CLV.
These examples illustrate the diverse and impactful ways in which predictive analytics can be integrated into various aspects of e-commerce operations to understand and enhance Customer Lifetime Value. By leveraging data-driven insights, e-commerce businesses can make more informed decisions, tailor their strategies to individual customer needs, and ultimately achieve greater profitability.
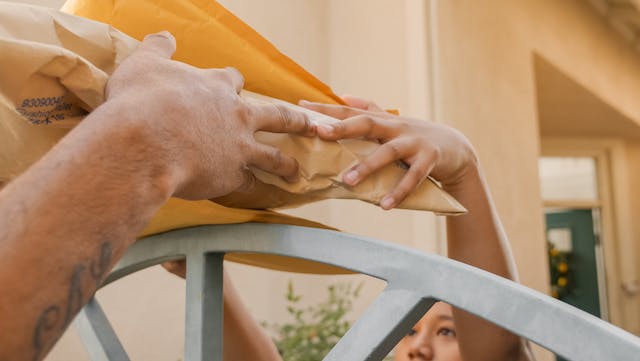
Conclusion
The potential of predictive analytics in enhancing Customer Lifetime Value (CLV) within the e-commerce sector is both substantial and transformative. By leveraging the vast amounts of data generated in digital transactions, predictive analytics offers deep insights into customer behavior, preferences, and future buying patterns. This enables e-commerce businesses to not only understand their customers more intimately but also to anticipate their needs and tailor their offerings accordingly.
The integration of predictive analytics into CLV calculations allows for a more nuanced and dynamic approach to customer relationship management. It goes beyond traditional metrics, providing a forward-looking perspective that is essential in today’s fast-paced market. E-commerce companies can benefit from personalized marketing strategies, optimized pricing models, effective customer segmentation, and improved customer retention, all of which contribute significantly to increasing the lifetime value of customers.
Furthermore, predictive analytics plays a crucial role in strategic decision-making. It enables businesses to allocate resources more efficiently, focus on the most profitable customer segments, and adapt strategies in real-time based on actionable insights. This data-driven approach leads to more informed decisions, reducing guesswork and enhancing the overall effectiveness of business strategies.
Therefore, it is highly encouraged for e-commerce businesses, regardless of their size, to adopt predictive analytics in their operations. The ability to predict future trends and customer behaviors can provide a significant competitive advantage. It empowers businesses to stay ahead of market trends, meet customer expectations more effectively, and ultimately drive sustainable growth and profitability.
The integration of predictive analytics into e-commerce strategies represents a major leap forward in how businesses understand and maximize customer lifetime value. It’s an investment in understanding the evolving landscape of customer needs and behaviors, and a commitment to responding with agility and precision. For any e-commerce business aiming to thrive in the digital age, embracing predictive analytics is not just an option, but a necessity for continued success and growth.
References
We can guide you on the types of sources you could seek out and where to find them for your research on predictive analytics in e-commerce and Customer Lifetime Value (CLV). Here are suggestions for types of sources and where you might locate them:
Scholarly Articles on Predictive Analytics and CLV:
Search academic databases like Google Scholar (scholar.google.com), JSTOR (www.jstor.org), or PubMed (for more scientific articles, at www.ncbi.nlm.nih.gov/pubmed).
Look for articles in journals specializing in data science, e-commerce, marketing, and business analytics.
Industry Reports on E-commerce and Predictive Analytics:
Websites of market research firms like Gartner (www.gartner.com), Forrester (www.forrester.com), and McKinsey & Company (www.mckinsey.com) often have reports on the latest trends in e-commerce and analytics.
You can also check out specialized e-commerce and retail research from sources like eMarketer (www.emarketer.com) and Nielsen (www.nielsen.com).
Books and eBooks:
Look for titles relating to e-commerce, predictive analytics, and customer relationship management on platforms like Amazon (www.amazon.com) and Google Books (books.google.com).
University libraries and online academic resources may also have relevant texts.
Online Courses and Educational Resources:
Websites like Coursera (www.coursera.org) and edX (www.edx.org) offer courses that cover predictive analytics and its application in e-commerce.
Often these courses provide additional reading materials and references.
Case Studies and White Papers:
Many business schools and companies publish case studies on the practical application of predictive analytics in e-commerce. Check the websites of business schools or look for white papers published by companies in the analytics field.
Technology and Data Science Blogs:
Blogs from companies specializing in analytics and data science, like SAS (blogs.sas.com), IBM Big Data & Analytics Hub (www.ibmbigdatahub.com), or Tableau (www.tableau.com/about/blog), often discuss relevant topics.
Remember, when referencing these materials in your article, it’s important to cite them properly to give credit to the original authors and to lend credibility to your work.
Frequently Asked Questions (FAQs) About Customer Lifetime Value in E-commerce
Here are some frequently asked questions (FAQs) about Customer Lifetime Value (CLV) in e-commerce, which address common queries and provide a basic understanding of this crucial metric:
What is Customer Lifetime Value (CLV) in E-commerce?
CLV is the total revenue a business can expect from a customer throughout their entire relationship. It’s a prediction of the net profit attributed to the entire future relationship with a customer.
Why is CLV important in E-commerce?
CLV is important because it helps businesses understand the long-term value of their customer relationships, enabling them to make informed decisions on marketing spend, customer acquisition, and retention strategies. It’s crucial for optimizing profitability and guiding resource allocation.
How is CLV calculated in E-commerce?
CLV can be calculated using several methods, but a common formula involves multiplying the average purchase value by the average purchase frequency rate and the average customer lifespan.
Can CLV predict the success of an E-commerce business?
While CLV is a powerful metric, it’s just one of many indicators of business health. A high CLV suggests strong customer loyalty and potential for sustained revenue, but it needs to be considered alongside other metrics like customer acquisition cost (CAC), conversion rate, and overall market trends.
How can E-commerce businesses increase CLV?
Businesses can increase CLV by enhancing customer experiences, offering personalized products or services, implementing loyalty programs, and maintaining high-quality customer service. Effective use of data analytics for targeted marketing also plays a crucial role.
What role does predictive analytics play in CLV?
Predictive analytics helps in forecasting future customer behavior, enabling businesses to tailor their strategies to maximize CLV. It involves using historical data to predict how long customers will stay and how much they will spend over time.
Is CLV equally important for all types of E-commerce businesses?
While CLV is a valuable metric for most e-commerce businesses, its importance can vary depending on the business model. For subscription-based models or businesses with a strong focus on repeat purchases, CLV is typically more critical.
How often should E-commerce businesses calculate CLV?
CLV should be monitored regularly, as it can change with market trends, customer behavior shifts, and as the business grows. Many businesses calculate CLV quarterly or annually, but the frequency can vary based on the specific needs and dynamics of the business.
Does improving CLV always mean reducing customer acquisition costs?
Not necessarily. While improving CLV can often lead to reduced acquisition costs (as more value is derived from each customer), the primary focus of CLV enhancement is on increasing the value obtained from customers over time, rather than just reducing upfront costs.
Can small E-commerce businesses effectively use CLV?
Absolutely. CLV is a valuable metric for businesses of all sizes. Smaller businesses can use CLV to focus their limited resources on the most profitable customer segments and tailor their strategies for long-term success.
Understanding these FAQs about CLV can provide e-commerce businesses and stakeholders with a foundational perspective on the importance and application of this key metric in driving growth and profitability.